For the last several years, we’ve been hearing about how much it costs to build ever larger language models. Today, a state-of-the-art language model requires approaching a million-trillion-trillion (1024) arithmetic operations involving hundreds of billions of parameters. Doing the math, assuming a decent, if older GPU, such as an A100, you come up with how many years this computation will take. Then you figure out how many GPUs you need given how many days you have to complete the computation. For example, Meta recently published that training a 65 billion parameter version of the LLaMA model using over a trillion tokens of text took approximately 21 days on roughly 2,000 such GPUs. That’s almost exactly 1 million hours of GPU time, which can be had for less than $1,000,000.
So, for $1 million, given a few decent machine learning folks, you could replicate a state-of-the-art language model or build your own tweaked to perform better in your domain, such as Bloomberg has done given its financial market. Expect to see much more of this from many corners, especially in healthcare, various areas of the life sciences, and others.
I would like to save the $1 million and start with the 30 or 65 billion parameter LLaMA model rather than train it from scratch. Unfortunately, Meta is not forthcoming with model weights for LLaMA beyond 13 billion parameters. The 13 billion parameter model is impressive enough. The 7B model is not capable enough for me. The 65 billion parameter model would be better, but not twice as good. The 30B parameter model is in the sweet spot.
Note that if you’re fine with a smaller pre-trained language mode, you could try Stability AI’s LM. These are the folks who brought you Stable Diffusion. They promise to eventually release, for any use including commercial, language models up to 65B. When available, the 15B model may be a good option. For now, I’d like to stick with LLaMA because of some of its significant algorithmic improvements.
Although available, even the 13 billion parameter model is not openly licensed. As is frustratingly common, the model weights are licensed only for research, not for commercial purposes. Meta invites commercial inquiry but, regrettably, based on my experience, is not eager to respond. So, wanting to use LLaMA commercially, you may have to train your own model. So, let’s talk budgets?
Training a 13 billion parameter model will cost about 20% of training a 65-billion parameter version. That will cost less than $200,000. You might be able to cut that cost by half, maybe more, however. It’s a little dicey, but you can cut back on the training data. Google’s excellent results from Chinchilla teach us to balance model size with training. OK, but the truth is that you can get over 90% of a language model’s final performance with less than half a trillion tokens of training data.
If you can afford it, you can avoid cutting back on the training data by taking your time. Your language model will be pretty good in 30% of the time Meta took and you can just let it improve over time. That is, start using the model and keep training it, replacing the one you’re using every once and a while. This is viable even if you perform fine-tuning (and even reinforcement learning) because the relative costs for such tuning are quite small versus pre-training.
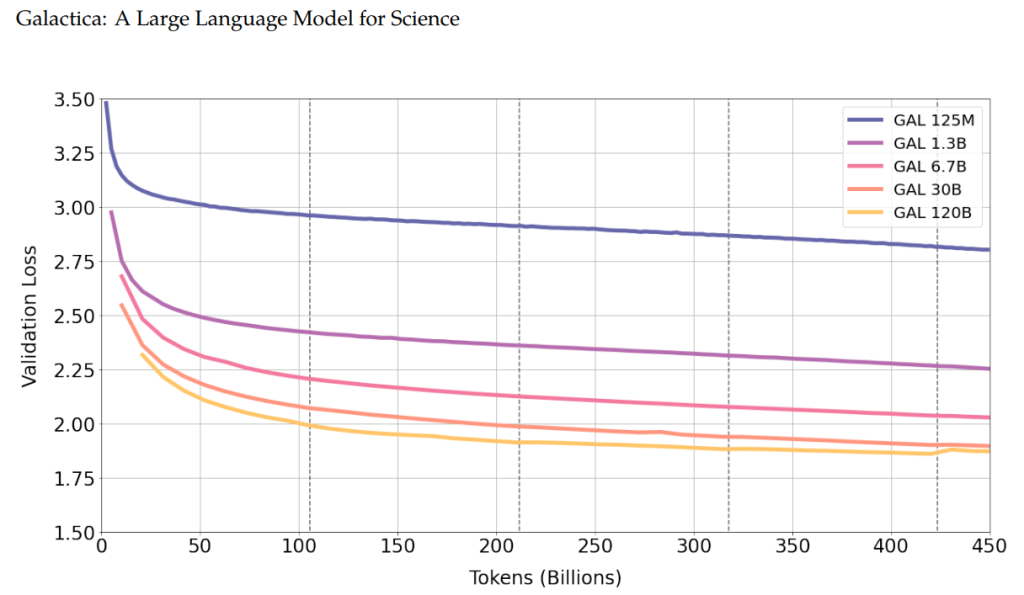
The bottom line here is that you can build your own 13 billion parameter LLaMA for less than $100,000. If you’re going to do millions of transactions, you might not be able to afford not to go in this direction!
LLaMA is essentially an improved version of Open AI’s GPT. LLaMA benefits from various algorithmic improvements since GPT-3 was released a couple years ago. Recently, Open AI introduce Instruct GPT, which follows instructions and Chat GPT, which holds conversations. And GPT has advanced to version 4.
LLaMA is GPT without the instruction following or conversational abilities. These are easy, and inexpensive, to add, however. Consider instruction following, for example. Researchers from Stanford generating tens of thousands of simple instructions and results using GPT-4 and trained the 7 billion parameter version of LLaMA with them. The dataset is relatively simple, and I thought weak, but was remarkably effective. I was quite surprised how well it follows instructions given only that simple, synthetic dataset.
On the other hand, it’s not all that surprising, given that we have seen much transfer of learned representations in vision. The ease of improvement here is simply because any decent generative language model will quickly adapt to using its representation to new linguistic sequences, such as those involving instructions. It doesn’t have to construct much new representation to do so.
The resulting language model is dubbed Alpaca; a cute play on words. Well, now there is Vicuna! Vicuna takes the 13 billion parameter LLaMA to approach ever more closely to Open AI’s state-of-the-art performance. According to the researchers from CMU, Stanford, and the University of California at Berkley and San Diego.
Look them up. It’s stunning how easily they compete with Google and approach Open AI. And the training cost to improve LLaMA to “within 10%” of GPT was less than $1,000. More and more is happening on this front. For more, see Microsoft’s DeepSpeed-Chat (which may seem odd given their investments in OpenAI, the company.).